S01E09 Cybernetics and the scientific discovery of feedback
Norbert Wiener, the inclusion of feedback in science, roots of complexity theory, biomimicry and biomimetics
In last week’s episode, it became clear that nature controls its systems through feedback. We also noted that human engineers didn’t catch up to the trick of automated control until the Industrial Revolution. In this episode, I explore where this relatively newfound control mechanism is leading us.
Mathematician and polymath Norbert Wiener was one of the first scientists to theorize that all intelligent behavior results from feedback mechanisms. He was a central figure in the origin story of complexity theory.
Norbert Wiener started in the field of control theory but extended it beyond engineering and mathematics. Early in his career, Wiener formulated a mathematical description of Brownian motion – the random movement of microscopic particles suspended in a gas or liquid. This breakthrough would allow him to predict the trajectory of these particles probabilistically.
Wiener spent World War II applying his theoretical work toward the war effort. More specifically, he worked on the problem of anti-aircraft artillery: how do you hit a target that is moving at high speed, high altitude, and that is actively trying to avoid being shot at? By WWII, planes were flying almost as fast as the payload of the AA guns targeting them, and gunners could no longer simply point the gun at the enemy. The plane would be long gone by the time its shells had arrived at that location.
Wiener came up with a computation device that translated the radar information of the target’s distance, direction, and speed into a prediction of the enemy aircraft’s flight path. This information was then fed back into the system, allowing the artillery to adjust its aim and fire at the predicted location of the aircraft. This feedback loop allowed the system to continuously update its predictions and improve its accuracy over time.
Wiener’s device started every computation with a new data set, unique to that pilot and plane. In computer science parlance, we say that the computation happens at runtime. Variables like the plane's capabilities, wind speeds, and the pilot’s temperament were all abstracted away into a time series of radar data. Wiener’s algorithms could then translate that data into a setpoint for the anti-aircraft system: a position of the anti-aircraft gun barrel that would blow the enemy out of the air:
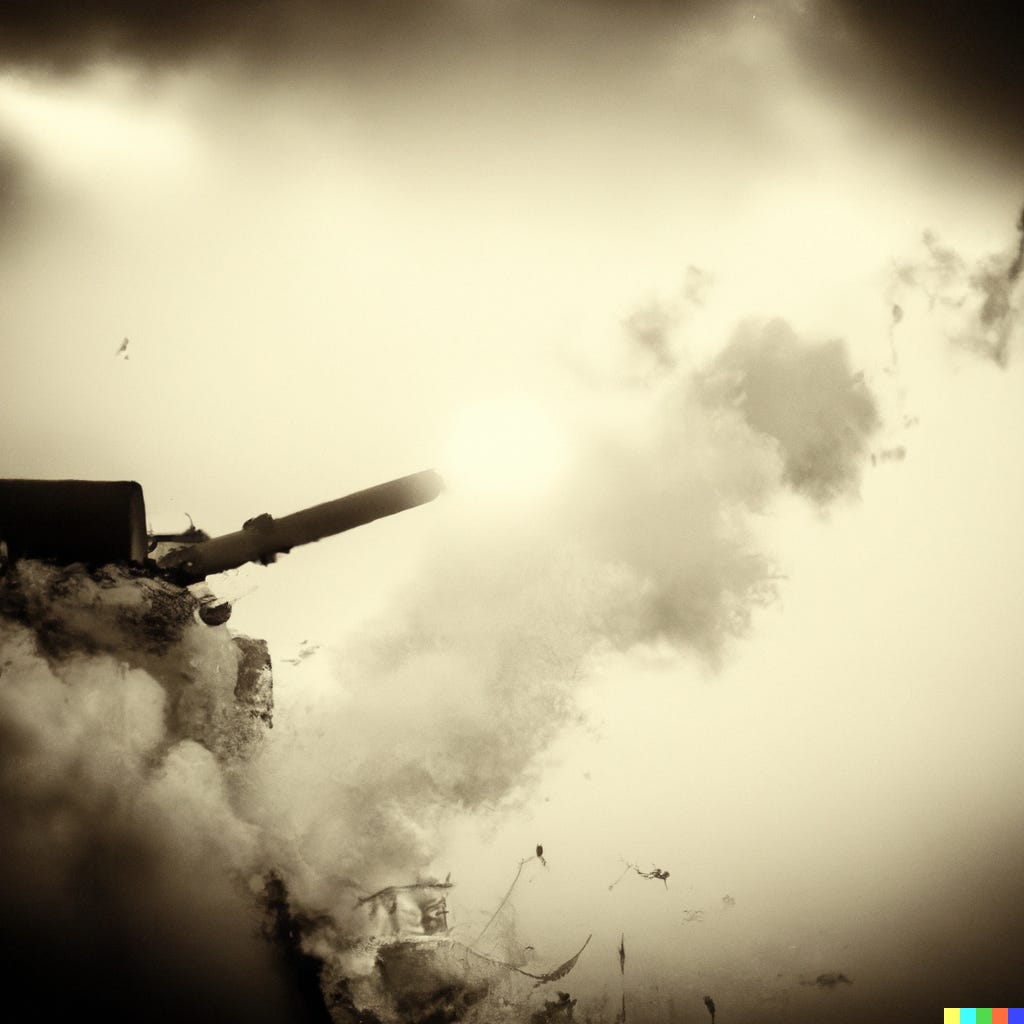
The wide applicability of self-correcting systems was not lost on Wiener, who was more drawn to developing the underlying theory than furthering military applications. Wiener grew convinced that the study of complexity should not focus on traditional physics concepts, such as mass and energy, but rather on concepts like feedback and information processing.
He called this new domain cybernetics, derived from the Greek word for steersman. Wiener summarized cybernetics as “the entire field of control and communication theory, whether in the machine or in the animal.” Controlling systems through feedback is now commonplace, and it’s hard for us to appreciate the reach of Wiener’s insights.
Wiener’s ideas quickly spread through the academic world. A group of scientists coalesced in the famous Macy conferences. These interdisciplinary meetings rallied scientists from domains as diverse as physics, psychology, biology, and computer science. Its participants were interested in finding common principles underlying complex systems and were generally fascinated by the analogies between the born and the made.
While cybernetics has not succeeded in formulating a unified information theory for feedback, its influence on science has been profound regardless. Thanks in large part to its interdisciplinary nature, cybernetics has managed to question many long-held scientific assumptions.
In a deterministic view of science, relative and probabilistic statements held little value. Thanks to Wiener and the people who followed in his footsteps, we can reason about systems in terms of circular causality, intentionality, feedback, hierarchies, and decentralized control.
In engineering, cybernetics would pave the way for countless industrial applications, from simple brakes and control circuits to complex systems like robotics and spacecraft propulsion. But cybernetics had more to contribute. Wiener’s definition of the field was novel in 2 ways: it extended control theory to communication (and therefore to information theory), and it did away with the distinction between man-made and living systems.
Cybernetics opened the door for technology to become more biological and for biology to become more technological. The idea of a brain-machine interface was first envisioned by Wiener himself in the 1940s. Advances in machine learning, robotics, and materials science have since led to proofs-of-concept of augmented human abilities.
Medical applications like the control of prosthetic limbs show that the brain-machine interface is no longer hypothetical. Elon Musk, the entrepreneur that is as prolific as he is controversial, is the co-founder of Neuralink, a company that is developing a chip that could be implanted in a person's brain. Neuralink also develops a robot to make the chip insertion process easier:
This will undoubtedly remind the reader of dystopian Hollywood movies, but cybernetics is not just about adding silicon concepts to biology. Technology is also being remodeled based on life.
Deep learning - a subset of machine learning - is fashioned after the way the human brain works. At its core, it uses a type of algorithm called an artificial neural network. These networks are comprised of small units called neurons that are connected together in layers.
Unlike traditional algorithms, which are explicitly programmed to perform a specific task, a deep learning model is trained using a large dataset of examples. The training process adjusts the connections between the neurons, known as weights, to optimize the model's performance on the task.
The key insight in the field of deep learning is that by increasing the size of the dataset and the complexity of the neural network, it is possible to achieve remarkable results, such as Copilot, Midjourney, and ChatGPT.
Another, lesser-known lineage of cybernetics led to fields like biomimetics and biomimicry. These fields apply principles from cybernetics to create technologies and systems that are able to adapt and self-regulate in response to their environment, just like living organisms do. For example:
self-healing concrete that can repair cracks and damage over time
solar panels that mimic the way leaves capture sunlight
designing enhanced materials based on naturally occurring properties like hydrophobicity or antibacterial properties
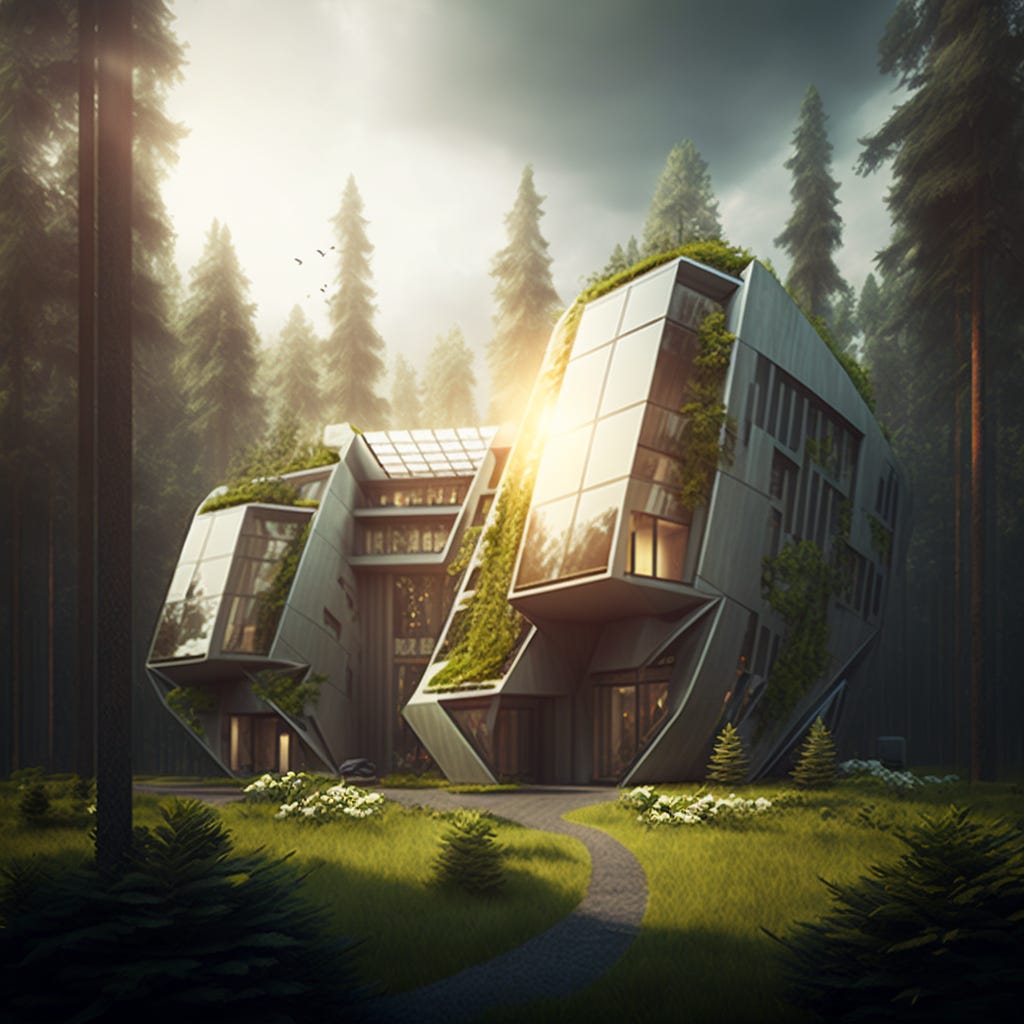
When it comes to management and organization, there have been a few attempts to create new models based on the principles of feedback, control, and biomimicry. Before jumping to that cybernetic branch, I want to explore another question that can help us understand the implications of feedback in organizational systems. Is there any way we can formally describe the feedback loops in a system? Can feedback help us create better models of complex systems?