S01E05 Recognizing complexity
Properties of complex systems: emergence, adaptation, signaling, information processing, non-linearity
Last week I suggested the complexity worldview could replace the modernist, mechanistic perspective. In this episode, I want to explore the properties that complex systems share.
Emergence & adaptation
A colony of ants is physically very different from a forest, an immune system, or an economy, but they all share an important trait. Every one of these systems exhibits complex collective behavior that emerges from the individual, interconnected components (ants, trees, cells, market participants). Their interactions give rise to complex and unpredictable behavior on a higher level. Our immune systems attack cancer cells, forests allocate soil resources, and economies enable price discovery.
Emergence enables the system’s ability to adapt. It is what sets complex systems apart from merely complicated systems like clockwork. Being adaptive means these systems can change their behavior to improve their chances of success. Adaptation can happen quickly, as is the case with a flock of birds in an evasive maneuver, or it can take place over long periods of time, as with human evolution.
Anthropologist Richard Wrangham argues that the human ability to cook food was the key factor in our evolutionary success. The newly emerged collective behavior of cooking made it easier for the human body to digest food. This was not just a competitive advantage vis-à-vis animals, but it also set off an evolutionary feedback loop that freed up energy for our brains to grow. Humans had learned to outsource parts of their metabolism to technology.
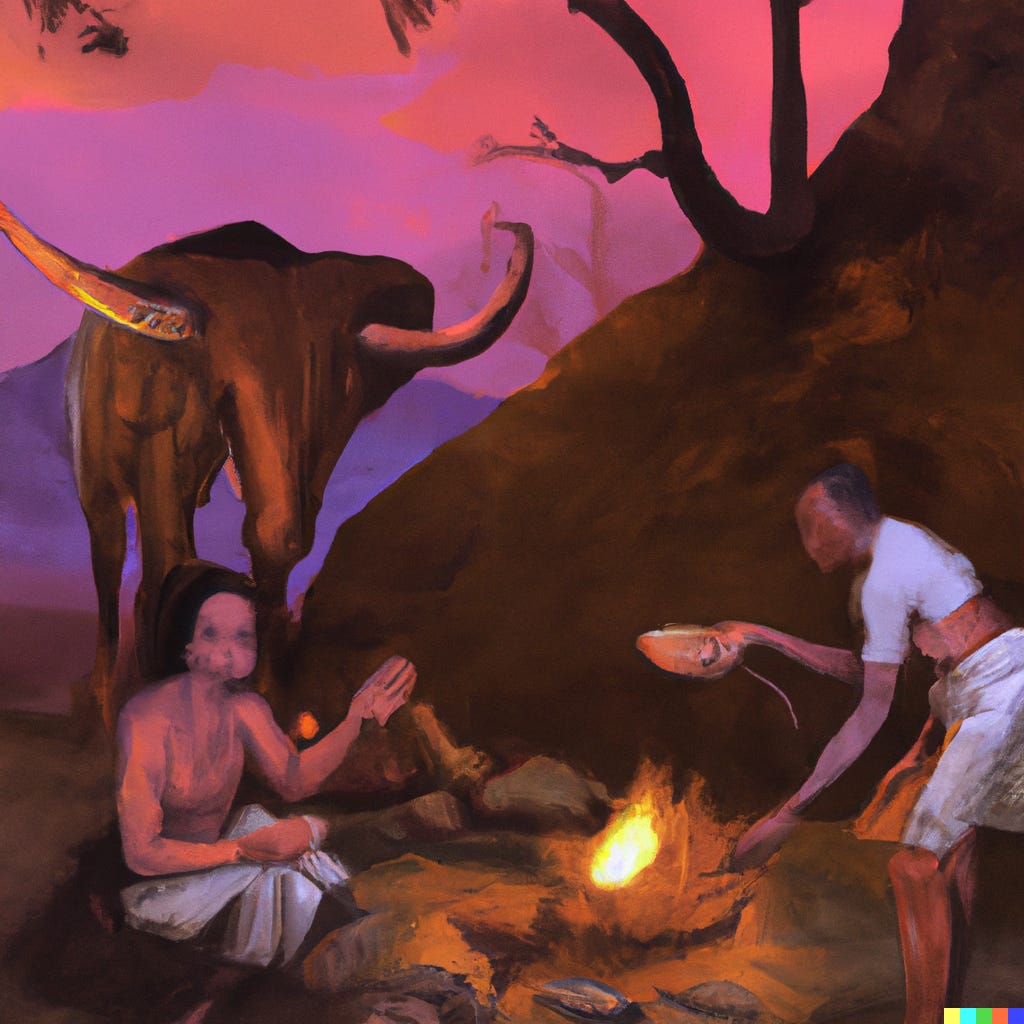
Technological progress only accelerated from there on out. Humans have since created technology that enables new kinds of complex, adaptive systems. Consider the Internet. This network wasn’t designed with any emergent, adaptive traits in mind but evolved them nonetheless. Systems researchers identified coevolutionary relationships between the different parts of the system like web pages and hyperlinks… As a result, the way information propagates over hyperlinks is adaptive.
To illustrate this evolution, think of the early days of the world wide web when the Internet was a network of read-only static web pages. The first major adaptation was so-called “user-generated” content, increasing interaction between participants. The web is currently undergoing another paradigm shift that revolves around concepts like encryption, ownership, permission, and identity.
The physicist Murray Gell-Mann said of complex adaptive systems that “although they differ widely in their physical attributes, they resemble one another in the way they handle information.” Let’s explore how that happens.
Signaling and information processing
Complex adaptive systems interact through signaling and information processing between interdependent parts. We can understand the moving parts in any system from just 2 types of feedback loops: self-reinforcing or self-correcting feedback loops. If a change in a variable amplifies whatever is happening, we call it self-reinforcing or positive.
In ant colonies, individual ants that have identified a food source deposit a pheromone trail on their way back to the nest. When other workers pick up on these pheromones, they start to follow the trail to the food source, leaving behind a larger pheromone trail, etc.
For a more technological example of a reinforcing feedback loop: the larger the user base of iPhones, the more attractive the iOS platform becomes for developers. The more 3rd party apps the platform has to offer, the more Apple can grow the install base,...
Self-correcting or negative feedback loops counteract change and seek equilibrium. In the ant example, overcrowding at the food source or depletion of the food leads to fewer ants returning to the nest with food, which leads to a weakened pheromone signal, which leads to fewer ants following the trail.
Even when we have an inkling of the feedback loops in play, we tend to overestimate our understanding of the system. A linear view of the world keeps us from understanding the downstream effects of feedback loops, multiple interconnections, nonlinearities, and time delays between interactions.
Yellowstone is an example of the unintended consequences of human intervention in a complex system. In 1916 a government agency launched a program to eradicate wolves in the national park. As wolves disappeared, the elk population could rise unchecked, which led to soil erosion and reduced growth of willow and aspen. In turn, fewer trees caused the beaver population to shrink.
In ecological terms, this is called a trophic cascade. The ecosystem collapsed because people with a limited understanding of the interconnections removed a single component from the food chain. Yellowstone's extermination of the wolves serves as a cautionary tale about our limited understanding of complex systems. On a brighter note: the wolves have since been reintroduced.
Non-linearity
Complex systems are non-linear; seemingly small changes can have significant and often unintended consequences. Non-linearity also brought the American meteorologist and mathematician Edward Lorenz to complexity theory.
“Determinism was equated with predictability before Lorenz. After Lorenz, we came to see that determinism might give you short-term predictability, but in the long run, things could be unpredictable. That’s what we associate with the word ‘chaos.’ ”
In 1961, Lorenz was using an early computer to simulate weather patterns. Lorenz’s weather model included a number of variables, representing things like temperature and wind speed. At one point, he wanted to re-check some conclusions and restarted the simulation halfway through to win time. To do this, he had to manually enter data from a printout corresponding to the conditions at that point in the simulation.
To Lorenz’s surprise, the simulation predicted entirely different weather than the initial simulation. As it turned out, the paper print-out used rounded numbers, so the numbers from the earlier simulation differed by a tiny fraction.
The effect of such a slight difference would be negligible in simpler, non-dynamical systems. In a complex system, this becomes impossible to predict. Scientists call this sensitive dependence on initial conditions. Here is a beautiful animation of how initial conditions can spiral out of control.
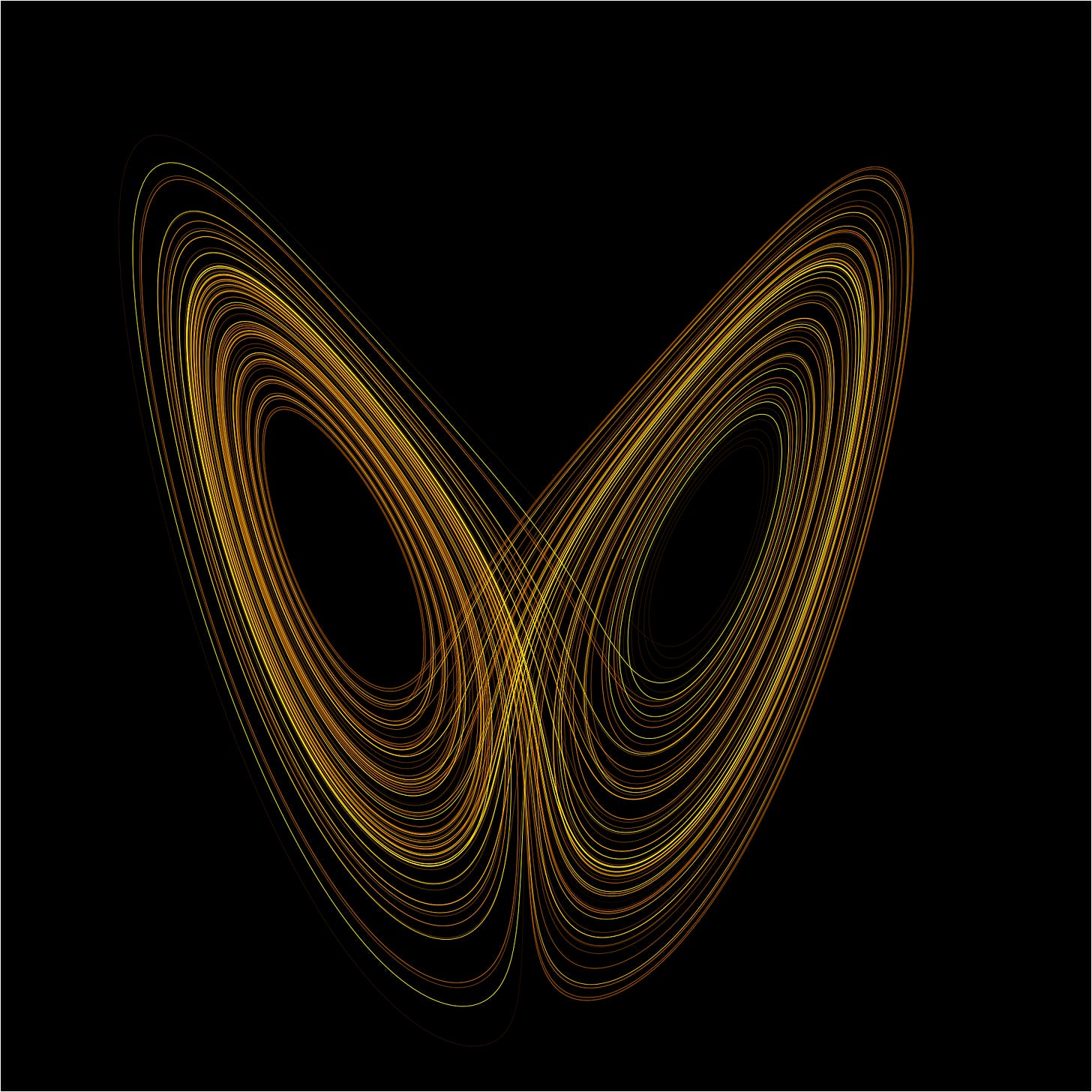
In a poetic turn of history, it seems that the name of the butterfly effect is not based on the visual similarity between Lorenz’s attractor and a butterfly but on the metaphor of a butterfly flapping its wings in Beijing, thereby causing rain in New York. How wonderfully strange that the graphical representation of this effect would also resemble a butterfly!
The butterfly effect is often misrepresented to make a point about leverage. It is not about tiny actions that can have big effects. The point is that the impact of an event in a complex system is not repeatable; we can’t predict what will happen the next time the butterfly flaps its wings (if anything).
This does not mean we should abandon all planning. Later in the season, I will tackle the implications of non-linear systems on organizational planning. Next episode, I’ll explore how information technology fits into the complexity worldview.
Further reading
Melanie Mitchell, Complexity: A Guided Tour